Enterprise Big Data
How can an enterprise leverage its legacy data while preparing for the deluge of new data sources?The Evolution of Data
Enterprise Big Data is a problem and an opportunity that permeates businesses and organizations alike. The problems arise when the volume or complexity of data grows to the point that tradition data processing applications such as relational databases, OLAP, and other technologies can no longer efficiently scale. At the same time, it is an opportunity in that all manner of unmeasured or unmeasurable activity has rapidly become tractable, opening the door to insights that eluded decision-makers a generation ago.
The demands of big data in the enterprise usually entail acquiring, curating, storing, searching, analyzing, maintaining and visualizing it. Technologies that assist in managing big data in the enterprise include parallel processing, unstructured storage, machine learning, natural language processing, enterprise search, cluster analysis, among others. Popular solutions have been built leveraging open source solutions such as Hadoop, which implements the MapReduce version of parallelism.
Rather than focus on solutions or technologies, enterprises should first consider fundamentals and whether or not enterprise big data has a role to play in addressing its challenges. We find that a useful framing device is to ask some fundamental questions about an Enterprise’s Data.
Questions to Ask about Enterprise Data
Organizations collect data for many reasons such as regulatory compliance, customer research, marketing and so on. In many cases, this data resides in standalone systems delivering value with relatively narrow impact. Across an enterprise, different groups could accumulate similar or even identical data about customers, processes, employees, and suppliers, competitors, operations, among many others. Some questions that become top of mind are:
- Is there a holistic view across all or most of the data sets?
- What redundancy is there in the data?
- How consistent and clean is the data?
- Does the company know how to use that data to find big value and opportunities?
- Does the organization aspire to better decisions through data-driven insight?
- What kind of unasked questions can its data answer?
Thoughtful exploration of these questions can help organizations breakthrough to the next level of success.
The Four V’s of Data
Enterprise data comes from many sources. Some types of data are created by people, for instance customers, suppliers, distributors, fans, or others, through direct conversations, chat rooms, social media and more. These data sources typically produce unstructured data. Other data comes from tools, such as CRM software or a timesheet application. And even more data might come from sensors. These other sources usually produce structured data. Such diverse data types require scalable workflows and technologies to collect, cleanse, store & host and visualize it. Data once tamed in this way can produce business insights to drive improvements to sales, risks, performance, customer support, marketing and more.
According to Gartner, the management of these aspects of data is known as the four V’s: Volume, Variety, Veracity, Velocity.
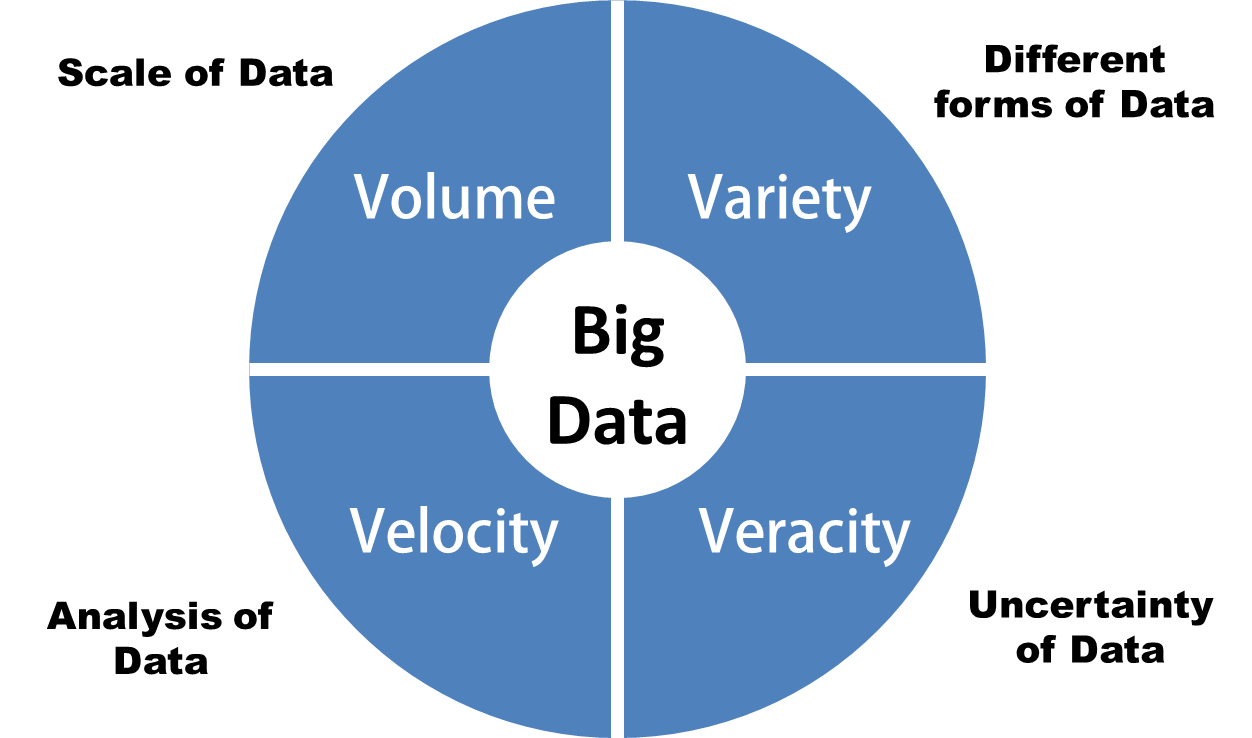
Volume
Often in the enterprise, the quantity of the data requires designing appropriate repositories to consume or manage that data with very tight performance or service level standards. In this respect, volume introduces challenges because technologies that would work with smaller data sets do not scale up. Methods and tools must be adapted to address the volume issues.
Variety
Some of that data might be unstructured (for example, raw text, Twitter feeds, audio, etc.), semi-structured or structured. In order to derive insights from it, the data must either be transformed to give it a coherent structure or managed in an entirely different way using unstructured approaches. Our data management consultants can help businesses with machine learning, cluster analysis techniques and more, to derive sense from unstructured data.
Veracity
Collecting data is increasingly automated, but there still are potential problems with the correctness of the data, such as quality, missing values, redundancy, pedigree, and so on. In most cases, it is necessary to develop processes and algorithms for data cleansing and enhancing data quality.
Velocity
Enterprise Data flows in streams that can increase and decrease. When the flow accelerates, Enterprise Data can cause challenges for systems to keep up with it. More importantly, as the velocity of data changes, the rate at which insights can be found should also change, allowing for faster response times.
Realizing the Full Value
Some organizations take advantage of their data from a traditional technology-centered approach. Today, most of data’s real value lies on the continuum of a human-centered approach. In that approach, real users are able to explore, filter and interact with data to discover patterns and understand why events, behaviors and other things happen in their business.
Dashboards are a stepping stone that introduces the organization to analytics and visualizations. In many cases, more important than routine dashboards, exploratory visualizations (a.k.a., “what-if” analysis) answer questions that cannot be found using other analytics or tools. Through exploratory visualizations it is easier to identify patterns that are otherwise hidden and to discover data problems and outliers.
We can help you with these issues and more.
LCL helped us develop tools to better present the data we had been collecting for years. In just a few months, they designed and delivered a solution that yielded new insights and allowed us to view the data in ways we couldn’t before. The visualizations they designed have led to new ideas both in presenting our data and for the data products we offer.